No. |
Title |
Authors |
Journal |
55 |
In silico re-identification of properties of drug target proteins |
27. Kim, B., Jo, J., Han, J., Park, C., Lee, H. |
BMC Bioinformatics. (2017) 18(SUPPLEMENT7): 248 |
Abstract
Background: Computational approaches in the identication of drug targets are expected to reduce time and effort in drug development. Advances in genomics and proteomics provide the opportunity to uncover properties of druggable genomes. Although several studies have been conducted for distinguishing drug targets from non-drug targets, they mainly focus on the sequences and functional
roles of proteins. Many other properties of proteins have not been fully investigated.
Methods: Using the DrugBank (version 3.0) database containing nearly 6,816 drug entries including 760 FDA-approved drugs and 1,822 of their targets and human UniProt/Swiss-Prot databases, we dened 1,578 non-redundant drug target and 17,575 non-drug target proteins. To select these non-redundant protein datasets, we built four datasets (A, B, C, and D) by considering clustering of paralogous proteins.
Results: We rst reassessed the widely used properties of drug target proteins. We conrmed and extended that drug target proteins (1) are likely to have more hydrophobic, less polar, less PEST sequences, and more signal peptide sequences higher and (2) are more involved in enzyme catalysis, oxidation and reduction in cellular respiration, and operational genes. In this study, we proposed new
properties (essentiality, expression pattern, PTMs, and solvent accessibility) for effectively identifying drug target proteins. We found that (1) drug targetability and protein essentiality are decoupled, (2) druggability of proteins has high expression level and tissue specicity, and (3) functional post-translational modication residues are enriched in drug target proteins. In addition, to predict the drug targetability of proteins, we exploited two machine learning methods (Support Vector Machine and Random Forest). When we predicted drug targets
by combining previously known protein properties and proposed new properties, an F-score of 0.8307 was obtained.
Conclusions: When the newly proposed properties are integrated, the prediction performance is improved and these properties are related to drug targets. We believe that our study will provide a new aspect in inferring drug-target interactions.
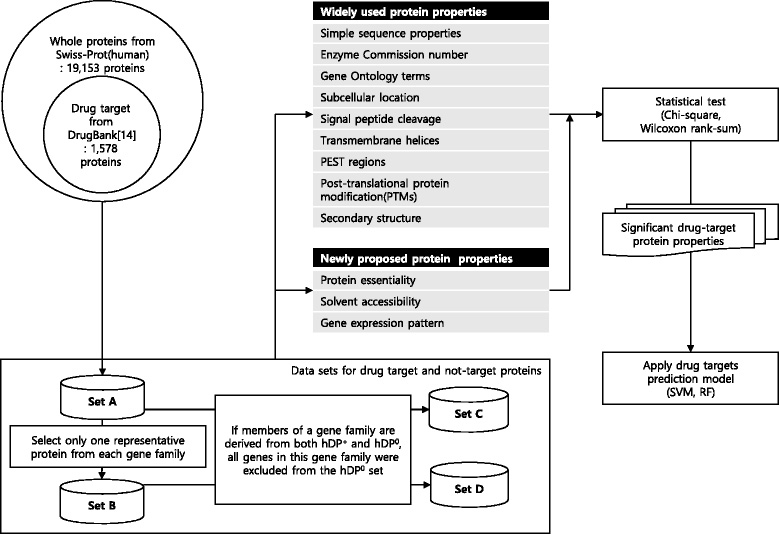